

A new measure for community structures through indirect social connections. Generation of uncorrelated random scale-free networks. In International Conference on Ubiquitous Computing and Ambient Intelligence, Springer, (pp.

Social network analysis applied to recommendation systems: alleviating the cold-user problem. Cambridge: MIT Press.Ĭastillejo, E., Almeida, A., & López-de Ipina, D. Microsoft Research.īriscoe, B., Odlyzko, A., & Tilly, B. Empirical analysis of predictive algorithms for collaborative filtering. New York: Springer Science & Business Media.īreese, J. Social networks and their economics: Influencing consumer choice. Cambridge: Cambridge University Press.īirke, D. Philosophical Transactions of the Royal Society A: Mathematical, Physical and Engineering Sciences, 371(1987), 20120375.īarabási, A. In Proceedings of the 21st international conference on World Wide Web, (pp. The role of social networks in information diffusion. Econometrica: Journal of the Econometric Society, 26, 1–23.īakshy, E., Rosenn, I., Marlow, C., & Adamic, L. Utilities, attitudes, choices: A review note. Rivista di diritto dei media, 2, 267–297.Īrrow, K. Economia della privacy: Una rassegna della letteratura (in italian). The Quarterly Journal of Economics, 84(3), 488–500.Īrpetti, J. The market for “Lemons” quality uncertainty and the market mechanism. Journal of Economic Literature, 54(2), 442–492.Īkerlof, G. IEEE Security and Privacy, 6(2), 46–50.Īcquisti, A., Taylor, C., & Wagman, L. Identity management, privacy, and price discrimination. While the k-nn algorithm proposes a classification measuring the distance between similar items or users in order to suggest which of them are closer (in terms of the already purchased item or in terms of users brought together by similar purchase histories) from the targeted user, k-mean algorithms are designed to cluster items (and so individuals on the basis of their choices and purchase histories when they are “user-item”), suggesting to the targeted subject those items surrounding those already purchased by him/her, thus defining the center of the temporary cluster and suggesting the closest nodes (Paterek 2007 Katarya and Verma 2016).Īcquisti, A.

The two most well-known classification and clustering algorithms-used by CF-are, respectively, k-nearest neighbour algorithm ( k-nn) (not to be confused with the “Nearest Neighbour Degree”, \(k_\), which origins from the network science field and which we will use later in this paper) and k-mean clustering (Sarwar et al. We tested our measures on Amazons’ instances, obtaining evidence which confirm the relevance of information extracted from nodes’ neighbourhood in order to steer targeted users. Our measure takes into account the quality of the connections among nodes as the one of a hypothetical user in relation to its neighbourhood, detecting how users with a good neighbourhood-hence of a superior connections set-obtain better information. We therefore propose a measure called Network Information Patrimony, considering the amount of information available within the system and we look into how platforms could exploit data stemming from connected profiles within a network, with a view to obtaining competitive advantages.
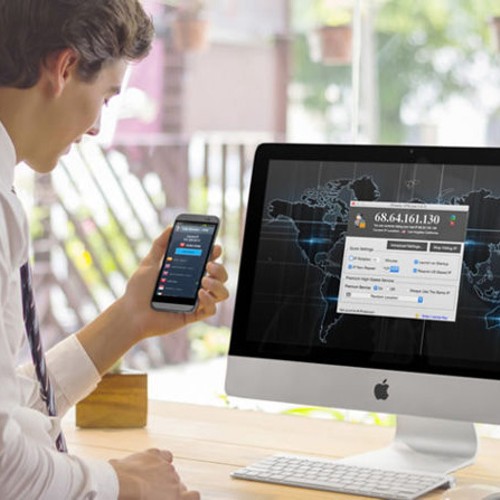
To this end, we rely on network science whose analytical tools allow data representations capable of highlighting relationships between subjects and/or items, extracting a great amount of information. In the present paper, we show how platforms’ performances still appear astonishing in relation to some unexplored data and networks properties, capable to enhance the platforms’ capacity to implement steering practices by means of an increased ability to estimate individuals’ preferences. Increased data gathering capacity, together with the spread of data analytics techniques, has prompterd an unprecedented concentration of information related to the individuals’ preferences in the hands of a few gatekeepers.
